Data Terms That Data Professional Should Know
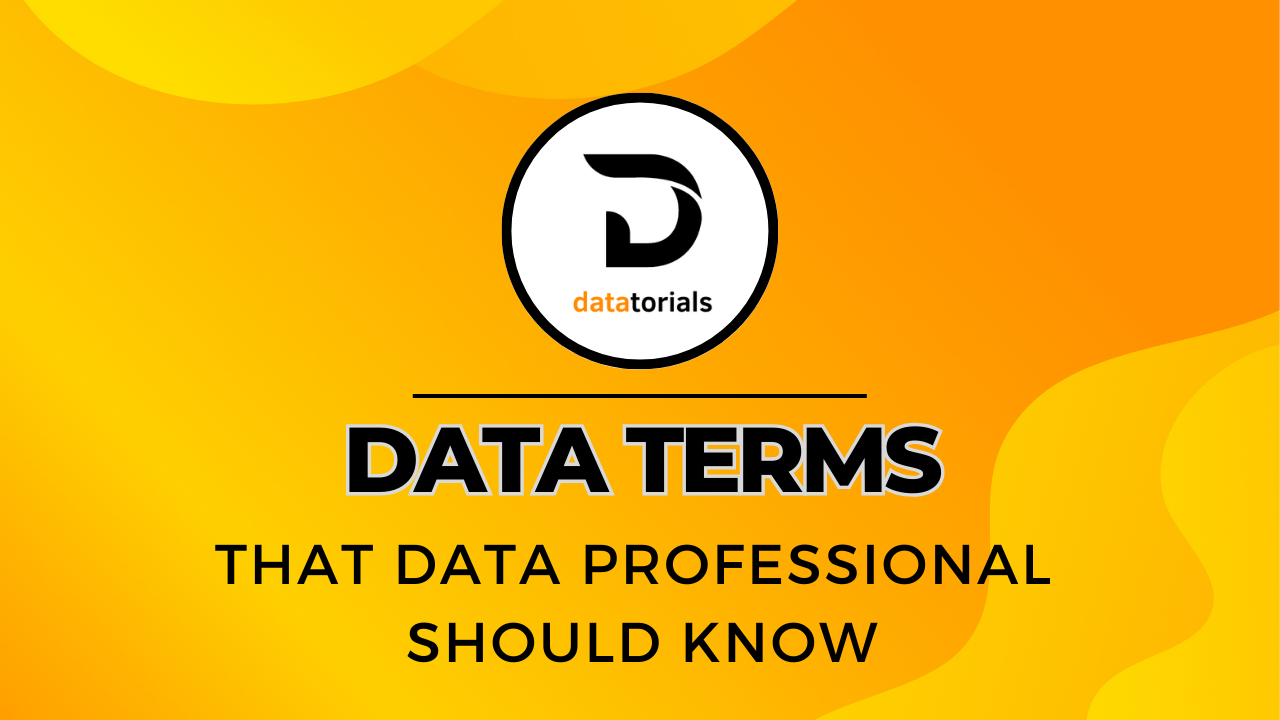
Data Warehouse:
Imagine a grand library of data that’s sorted and organized neatly for analysis and reporting. A data storehouse is just that. With its schema- on- write approach, it offers a structured layout – generally star schema or snowflake schema – which makes it perfect for querying literal data and business intelligence.
Data Mart:
Picture a corner store that’s part of a larger shopping complex. That’s what a data emporium is to a data storehouse. It’s a technical section serving the requirements of specific business units or brigades, speeding up data reclamation and analysis for the sphere it serves.
Data Lake:
A data lake is like an enormous force holding a different blend of raw data, both structured and unshaped. Because of its schema- on- read approach and its capability to store data in its native format, it’s a go- to for big data and machine literacy operations. Technologies like Hadoop, Apache Spark, and NoSQL databases help it process huge volumes of data efficiently. Delta Lake Consider Delta Lake as a safety subcaste over your data lake. It ensures data integrity with ACID sale support, allows literal data reclamation through data versioning, and manages small train issues with automatic contraction.
Data Pipeline:
Just as a shelter transports people from one place to another, a data channel moves data from its source to its destination. It uses ETL or ELT way to ingest, transfigure, and deliver data for colorful logical uses. Tools like Apache Beam, Airflow, and Kafka are vital in erecting effective channels.
Data Mesh:
This fresh approach sees data as a product, resolving scalability issues of monolithic infrastructures by decentralizing data power and armature. With this approach, brigades can tone- serve their data needs. Principles of microservices armature and sphere- driven design are crucial in enforcing a data mesh.
Data Lakehouse:
A data lakehouse is like a cold-blooded home that combines the stylish features of a data storehouse( structured querying capabilities) and a data lake( scalability and inflexibility). It uses technologies like Apache Spark and data formats like Parquet and Delta Lake to deliver BI and machine literacy capabilities from the same platform.
Data Swamp:
This is a exemplary tale of a data lake gone bad. Without proper operation, a data lake can turn into a data swamp, where data is disorganized, inapproachable, andnon-compliant, emphasizing the significance of proper data governance and quality control.
Data Fabric:
This is the underpinning structure designed to manage data end- to- end. It uses AI, machine literacy, and semantics to produce a dynamic system that can pierce, discover, transfigure, and integrate data across different sources, locales, and formats.