Ethical Considerations in Data Science: Balancing Innovation and Responsibility
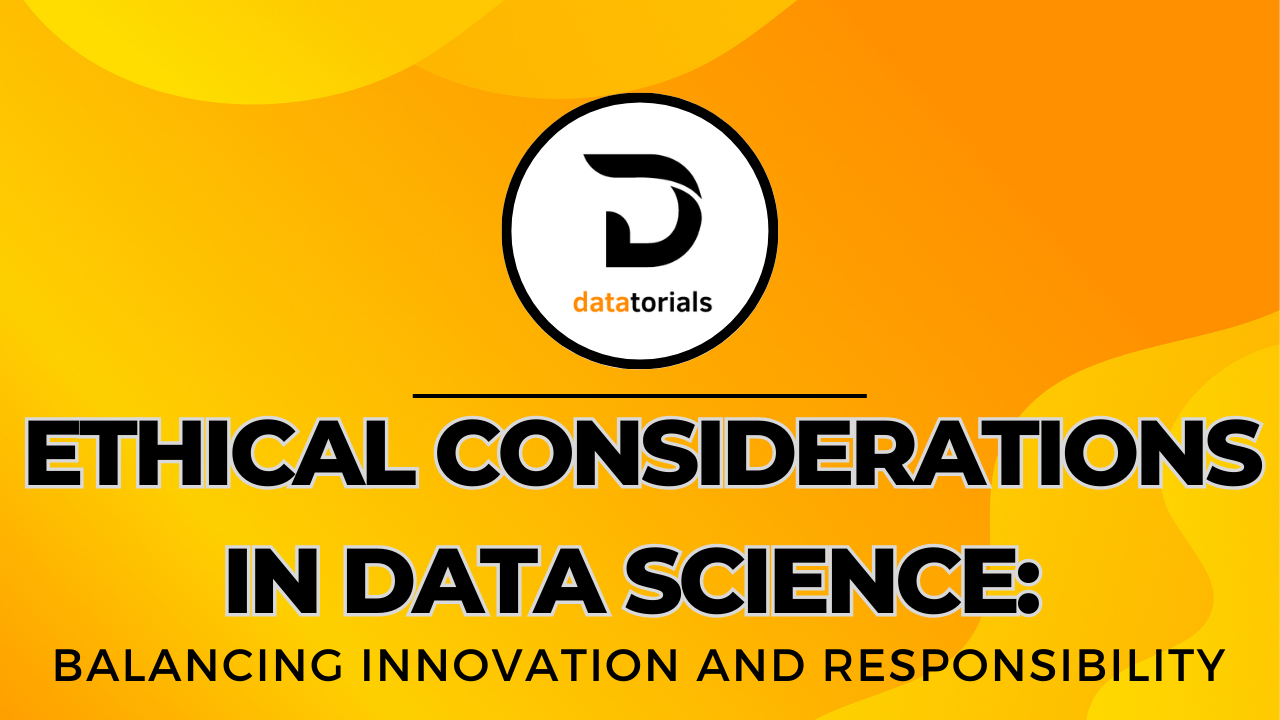
Introduction
The rapid growth of data science and its applications has ushered in an era of unprecedented opportunities and challenges. While data science has the potential to drive innovation and solve complex problems, it also raises important ethical considerations. In this article, we will explore the ethical dimensions of data science, highlighting the need for responsible practices and the potential consequences of unethical data handling.
The Power and Responsibility of Data Science
Data science is a powerful tool that can uncover valuable insights, automate decision-making processes, and drive business success. However, this power comes with a responsibility to ensure that data is handled ethically and responsibly. Here are some key ethical considerations in data science:
Privacy and Consent: One of the most fundamental ethical concerns is the protection of individuals’ privacy. Data scientists must obtain informed consent when collecting and using personal data. Transparency about data usage and the right to opt-out should be prioritized.
Bias and Fairness: Biases can inadvertently creep into algorithms and models, leading to unfair outcomes. Data scientists must be vigilant in identifying and mitigating bias to ensure fair and equitable results, especially in applications like hiring and lending.
Data Security: Safeguarding data from breaches and unauthorized access is paramount. Data encryption, access controls, and regular security audits are essential to protect sensitive information.
Accountability: Data scientists and organizations must be accountable for their actions. Clear guidelines and standards should be established to ensure ethical conduct throughout the data lifecycle.
Transparency: Transparent reporting of methods, data sources, and model decisions is crucial. This transparency builds trust with stakeholders and allows for scrutiny of results.
Case Studies in Ethical Dilemmas
To illustrate the ethical complexities of data science, let’s examine a couple of real-world case studies:
Predictive Policing: Predictive policing algorithms use historical crime data to allocate police resources. Critics argue that these algorithms may perpetuate bias by focusing resources on already over-policed communities, potentially exacerbating existing inequalities.
Social Media Manipulation: Social media platforms have faced scrutiny for their role in spreading misinformation and facilitating political manipulation. Ethical concerns center on algorithmic amplification of polarizing content and lack of transparency in content moderation.
The Path Forward: Responsible Data Science
Addressing the ethical challenges in data science requires a concerted effort from data scientists, organizations, and policymakers. Here are some steps toward responsible data science:
Ethical Education: Data scientists should receive training in ethical considerations and be aware of the potential biases and consequences of their work.
Ethical Frameworks: Organizations should adopt ethical frameworks and guidelines that prioritize transparency, fairness, and privacy in data science projects.
Collaboration: Collaboration between data scientists, ethicists, and domain experts can help ensure a multidisciplinary approach to ethical decision-making.
Regulatory Oversight: Policymakers should enact and enforce regulations that protect individuals’ rights and promote ethical data practices.
Conclusion
Data science has the power to transform industries and drive innovation, but it also comes with ethical responsibilities. As data continues to play a central role in our lives, it is essential that we navigate the ethical challenges with diligence and integrity. By fostering a culture of responsible data science, we can harness the benefits of this field while minimizing its potential harm and ensuring a more ethical and equitable future.